Model reference adaptive system speed estimator based on type-1 and type-2 fuzzy logic sensorless control of electrical vehicle with electrical differential
DOI:
https://doi.org/10.20998/2074-272X.2023.4.03Keywords:
electrical vehicle, induction machines, model reference adaptive system, field oriented control, electric differential, fuzzy logic controllerAbstract
Introduction. In this paper, a new approach for estimating the speed of in-wheel electric vehicles with two independent rear drives is presented. Currently, the variable-speed induction motor replaces the DC motor drive in a wide range of applications, including electric vehicles where quick dynamic response is required. This is now possible as a result of significant improvements in the dynamic performance of electrical drives brought about by technological advancements and development in the fields of power commutation devices, digital signal processing, and, more recently, intelligent control systems. The system’s reliability and robustness are improved, and the cost, size, and upkeep requirements of the induction motor drive are reduced through control strategies without a speed sensor. Successful uses of the induction motor without a sensor have been made for medium- and high-speed operations. However, low speed instability and instability under various charge perturbation conditions continue to be serious issues in this field of study and have not yet been effectively resolved. Some application such as traction drives and cranes are required to maintain the desired level of torque down to low speed levels with uncertain load torque disturbance conditions. Speed and torque control is more important particularly in motor-in-wheel traction drive train configuration where vehicle wheel rim is directly connected to the motor shaft to control the speed and torque. Novelty of the proposed work is to improve the dynamic performance of conventional controller used of model reference adaptive system speed observer using both type-1 and type-2 fuzzy logic controllers. Purpose. In proposed scheme, the performance of the engine is being controlled, fuzzy logic controller is controlling the estimate rotor speed, and results are then compared using type-1 and type-2. Method. For a two-wheeled motorized electric vehicle, a high-performance sensorless wheel motor drive based on both type-2 and type-1 fuzzy logic controllers of the model reference adaptive control system is developed. Results. Proved that, using fuzzy logic type-2 controller the sensorless speed control of the electrical differential of electric vehicle EV observer, much better results are achieved. Practical value. The main possibility of realizing reliable and efficient electric propulsion systems based on intelligent observers (type-2 fuzzy logic) is demonstrated. The research methodology has been designed to facilitate the future experimental implementation on a digital signal processor.
References
Zadeh L.A. Fuzzy sets. Information and Control, 1965, vol. 8, no. 3, pp. 338-353. doi: https://doi.org/10.1016/S0019-9958(65)90241-X.
Mizumoto M., Tanaka K. Some properties of fuzzy sets of type 2. Information and Control, 1976, vol. 31, no. 4, pp. 312-340. doi: https://doi.org/10.1016/S0019-9958(76)80011-3.
Karnik N.N., Mendel J.M. Applications of type-2 fuzzy logic systems: handling the uncertainty associated with surveys. FUZZ-IEEE’99. 1999 IEEE International Fuzzy Systems. Conference Proceedings (Cat. No.99CH36315), 1999, vol. 3, pp. 1546-1551 doi: https://doi.org/10.1109/FUZZY.1999.790134.
Mousavi S.M. A new interval-valued hesitant fuzzy pairwise comparison–compromise solution methodology: an application to cross-docking location planning. Neural Computing and Applications, 2019, vol. 31, no. 9, pp. 5159-5173. doi: https://doi.org/10.1007/s00521-018-3355-y.
Tsang Y.P., Wong W.C., Huang G.Q., Wu C.H., Kuo Y.H., Choy K.L. A Fuzzy-Based Product Life Cycle Prediction for Sustainable Development in the Electric Vehicle Industry. Energies, 2020, vol. 13, no. 15, art. no. 3918. doi: https://doi.org/10.3390/en13153918.
Seth A.K., Singh M. Unified adaptive neuro-fuzzy inference system control for OFF board electric vehicle charger. International Journal of Electrical Power & Energy Systems, 2021, vol. 130, art. no. 106896. doi: https://doi.org/10.1016/j.ijepes.2021.106896.
Williamson S.S., Rathore A.K., Musavi F. Industrial Electronics for Electric Transportation: Current State-of-the-Art and Future Challenges. IEEE Transactions on Industrial Electronics, 2015, vol. 62, no. 5, pp. 3021-3032. doi: https://doi.org/10.1109/TIE.2015.2409052.
Haddoun A., Benbouzid M.E.H., Diallo D., Abdessemed R., Ghouili J., Srairi K. Modeling, Analysis, and Neural Network Control of an EV Electrical Differential. IEEE Transactions on Industrial Electronics, 2008, vol. 55, no. 6, pp. 2286-2294. doi: https://doi.org/10.1109/TIE.2008.918392.
Yang J., Zhang T., Zhang H., Hong J., Meng Z. Research on the Starting Acceleration Characteristics of a New Mechanical–Electric–Hydraulic Power Coupling Electric Vehicle. Energies, 2020, vol. 13, no. 23, art. no. 6279. doi: https://doi.org/10.3390/en13236279.
Tao Guilin, Ma Zhiyun, Zhou Libing, Li Langru. A novel driving and control system for direct-wheel-driven electric vehicle. 2004 12th Symposium on Electromagnetic Launch Technology, 2004, pp. 514-517. doi: https://doi.org/10.1109/ELT.2004.1398134.
Ju-Sang Lee, Young-Jae Ryoo, Young-Cheol Lim, Freere P., Tae-Gon Kim, Seok-Jun Son, Eui-Sun Kim. A neural network model of electric differential system for electric vehicle. 2000 26th Annual Conference of the IEEE Industrial Electronics Society. IECON 2000. 2000 IEEE International Conference on Industrial Electronics, Control and Instrumentation. 21st Century Technologies and Industrial Opportunities (Cat. No.00CH37141), vol. 1, pp. 83-88. doi: https://doi.org/10.1109/IECON.2000.973130.
Han-Xiong Li, Gatland H.B. A new methodology for designing a fuzzy logic controller. IEEE Transactions on Systems, Man, and Cybernetics, 1995, vol. 25, no. 3, pp. 505-512. doi: https://doi.org/10.1109/21.364863.
Guezi A., Bendaikha A., Dendouga A. Direct torque control based on second order sliding mode controller for three-level inverter-fed permanent magnet synchronous motor: comparative study. Electrical Engineering & Electromechanics, 2022, no. 5, pp. 10-13. doi: https://doi.org/10.20998/2074-272X.2022.5.02.
Chekroun S., Abdelhadi B., Benoudjit A. Design optimization of induction motor using hybrid genetic algorithm «a critical analyze». Advances in Modelling and Analysis C, 2016, vol. 71, no. 1, pp. 1-23.
Naït-Saïd M.-S., Tadjine M., Drid S. Robust backstepping vector control for the doubly fed induction motor. IET Control Theory & Applications, 2007, vol. 1, no. 4, pp. 861-868. doi: https://doi.org/10.1049/iet-cta:20060053.
Young Ahn Kwon, Dae Won Jin. A novel MRAS based speed sensorless control of induction motor. IECON’99. Conference Proceedings. 25th Annual Conference of the IEEE Industrial Electronics Society (Cat. No.99CH37029), 1999, vol. 2, pp. 933-938. doi: https://doi.org/10.1109/IECON.1999.816537.
Khemis A., Benlaloui I., Drid S., Chrifi-Alaoui L., Khamari D., Menacer A. High-efficiency induction motor drives using type-2 fuzzy logic. The European Physical Journal Plus, 2018, vol. 133, no. 3, art. no. 86. doi: https://doi.org/10.1140/epjp/i2018-11903-6.
Benlaloui I., Drid S., Chrifi-Alaoui L., Ouriagli M. Implementation of a New MRAS Speed Sensorless Vector Control of Induction Machine. IEEE Transactions on Energy Conversion, 2015, vol. 30, no. 2, pp. 588-595. https://doi.org/10.1109/TEC.2014.2366473.
Zaky M.S., Khater M.M., Shokralla S.S., Yasin H.A. Wide-Speed-Range Estimation With Online Parameter Identification Schemes of Sensorless Induction Motor Drives. IEEE Transactions on Industrial Electronics, 2009, vol. 56, no. 5, pp. 1699-1707. doi: https://doi.org/10.1109/TIE.2008.2009519.
Vasic V., Vukosavic S. PES News. IEEE Power Engineering Review, 2001, vol. 21, no. 11, pp. 16-17. doi: https://doi.org/10.1109/39.961999.
Miloud Y., Draou A. Fuzzy logic based rotor resistance estimator of an indirect vector controlled induction motor drive. IEEE 2002 28th Annual Conference of the Industrial Electronics Society. IECON 02, vol. 2, pp. 961-966. doi: https://doi.org/10.1109/IECON.2002.1185402.
Ali Moussa M., Derrouazin A., Latroch M., Aillerie M. A hybrid renewable energy production system using a smart controller based on fuzzy logic. Electrical Engineering & Electromechanics, 2022, no. 3, pp. 46-50. doi: https://doi.org/10.20998/2074-272X.2022.3.07.
Bordons C., Ridao M.A., Perez A., Arce A., Marcos D. Model Predictive Control for power management in hybrid fuel cell vehicles. 2010 IEEE Vehicle Power and Propulsion Conference, 2010, pp. 1-6. doi: https://doi.org/10.1109/VPPC.2010.5729119.
Vaez S., John V.I., Rahman M.A. Energy saving vector control strategies for electric vehicle motor drives. Proceedings of Power Conversion Conference - PCC ’97, vol. 1, pp. 13-18. doi: https://doi.org/10.1109/PCCON.1997.645578.
Takeda K., Takahashi C., Arita H., Kusumi N., Amano M., Emori A. Design of hybrid energy storage system using dual batteries for renewable applications. 2014 IEEE PES General Meeting | Conference & Exposition, 2014, pp. 1-5. doi: https://doi.org/10.1109/PESGM.2014.6938860.
Colyer R.E., Economou J.T. Comparison of steering geometries for multi-wheeled vehicles by modelling and simulation. Proceedings of the 37th IEEE Conference on Decision and Control (Cat. No.98CH36171), 1998, vol. 3, pp. 3131-3133. doi: https://doi.org/10.1109/CDC.1998.757992.
Xia X., Xing Y., Wei B., Zhang Y., Li X., Deng X., Gui L. A fitness-based multi-role particle swarm optimization. Swarm and Evolutionary Computation, 2019, vol. 44, pp. 349-364. doi: https://doi.org/10.1016/j.swevo.2018.04.006.
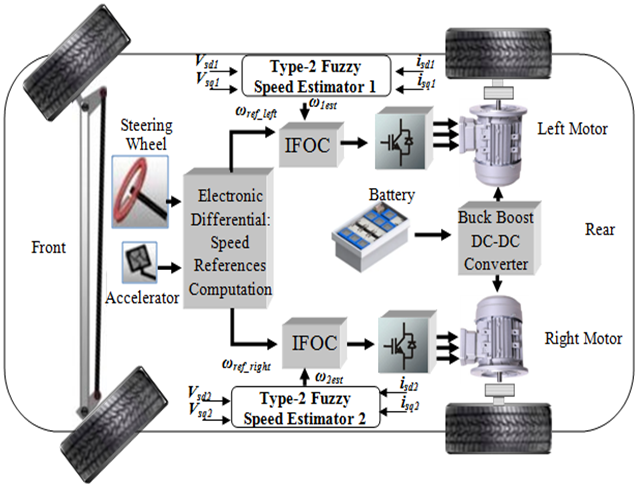
Downloads
Published
How to Cite
Issue
Section
License
Copyright (c) 2023 A. Khemis, T. Boutabba, S. Drid

This work is licensed under a Creative Commons Attribution-NonCommercial 4.0 International License.
Authors who publish with this journal agree to the following terms:
1. Authors retain copyright and grant the journal right of first publication with the work simultaneously licensed under a Creative Commons Attribution License that allows others to share the work with an acknowledgement of the work's authorship and initial publication in this journal.
2. Authors are able to enter into separate, additional contractual arrangements for the non-exclusive distribution of the journal's published version of the work (e.g., post it to an institutional repository or publish it in a book), with an acknowledgement of its initial publication in this journal.
3. Authors are permitted and encouraged to post their work online (e.g., in institutional repositories or on their website) prior to and during the submission process, as it can lead to productive exchanges, as well as earlier and greater citation of published work.