Power transformer faults diagnosis using undestructive methods (Roger and IEC) and artificial neural network for dissolved gas analysis applied on the functional transformer in the Algerian north-eastern: a comparative study
DOI:
https://doi.org/10.20998/2074-272X.2021.4.01Keywords:
analysis of dissolved gases in oil, diagnostics of power transformers, feed-forward neural networks, Rogers method, IEC methodAbstract
Introduction. Nowadays, power transformer aging and failures are viewed with great attention in power transmission industry. Dissolved gas analysis (DGA) is classified among the biggest widely used methods used within the context of asset management policy to detect the incipient faults in their earlier stage in power transformers. Up to now, several procedures have been employed for the lecture of DGA results. Among these useful means, we find Key Gases, Rogers Ratios, IEC Ratios, the historical technique less used today Doernenburg Ratios, the two types of Duval Pentagons methods, several versions of the Duval Triangles method and Logarithmic Nomograph. Problem. DGA data extracted from different units in service served to verify the ability and reliability of these methods in assessing the state of health of the power transformer. Aim. An improving the quality of diagnostics of electrical power transformer by artificial neural network tools based on two conventional methods in the case of a functional power transformer at Sétif province in East North of Algeria. Methodology. Design an inelegant tool for power transformer diagnosis using neural networks based on traditional methods IEC and Rogers, which allows to early detection faults, to increase the reliability, of the entire electrical energy system from transport to consumers and improve a continuity and quality of service. Results. The solution of the problem was carried out by using feed-forward back-propagation neural networks implemented in MATLAB-Simulink environment. Four real power transformers working under different environment and climate conditions such as: desert, humid, cold were taken into account. The practical results of the diagnosis of these power transformers by the DGA are presented. Practical value. The structure and specific features of power transformer winding insulation ageing and defect state diagnosis by the application of the artificial neural network (ANN) has been briefly given. MATLAB programs were then developed to automate the evaluation of each method. This paper presents another tool to review the results obtained by the delta X software widely used by the electricity company in Algeria.
References
Li Z., Jiao Z., He A. Knowledge-based artificial neural network for power transformer protection. IET Generation, Transmission & Distribution, 2020, vol. 14, no. 24, pp. 5782-5791. doi: https://doi.org/10.1049/iet-gtd.2020.0542.
Rozov V.Yu., Kundius K.D., Pelevin D.Ye. Active shielding of external magnetic field of built-in transformer substations. Electrical Engineering & Electromechanics, 2020, no. 3, pp. 24-30. doi: https://doi.org/10.20998/2074-272x.2020.3.04.
Bouchaoui L., Hemsas K.E., Benlahneche S.E. Diagnostic des transformateurs de puissance. France, Omniscriptum Publ., 2018. 210 p. (Fra).
Bondarenko V.Е., Shutenko O.V. Development of fuzzy neural network for the interpretation of the results of dissolved in oil gases analysis. Electrical Engineering & Electromechanics, 2017, no. 2, pp. 49-56. doi: https://doi.org/10.20998/2074-272x.2017.2.08.
Nizhevskyi I.V., Nizhevskyi V.I. Calculation estimation of overvoltage on insulation of the equipment of a substation at the lightning strike in its lightning arrester. Electrical Engineering & Electromechanics, 2019, no.3, pp. 67-73. doi: https://doi.org/10.20998/2074-272x.2019.3.11.
Faiz J., Soleimani M. Dissolved gas analysis evaluation in electric power transformers using conventional methods a review. IEEE Transactions on Dielectrics and Electrical Insulation, 2017, vol. 24, no. 2, pp. 1239-1248. doi: https://doi.org/10.1109/tdei.2017.005959.
Castro A.R.G., Miranda V. An interpretation of neural networks as inference engines with application to transformer failure diagnosis. International Journal of Electrical Power & Energy Systems, 2005, vol. 27, no. 9-10, pp. 620-626. doi: https://doi.org/10.1016/j.ijepes.2005.08.002.
Wani S.A., Khan S.A., Prashal G., Gupta D. Smart diagnosis of incipient faults using dissolved gas analysis-based fault interpretation matrix (FIM). Arabian Journal for Science and Engineering, 2019, vol. 44, no. 8, pp. 6977-6985. doi: https://doi.org/10.1007/s13369-019-03739-4.
Faiz J., Soleimani M. Assessment of computational intelligence and conventional dissolved gas analysis methods for transformer fault diagnosis. IEEE Transactions on Dielectrics and Electrical Insulation, 2018, vol. 25, no. 5, pp. 1798-1806. doi: https://doi.org/10.1109/TDEI.2018.007191.
Kaur A., Brar Y.S., Leena G. Fault detection in power transformers using random neural networks. International Journal of Electrical and Computer Engineering, 2019, vol. 9, no. 1, pp. 78-84. doi: https://doi.org/10.11591/ijece.v9i1.pp78-84.
Zhang Y., Ding X., Liu Y., Griffin P.J. An artificial neural network approach to transformer fault diagnosis. IEEE Transactions on Power Delivery, 1996, vol. 11, no. 4, pp. 1836-1841. doi: https://doi.org/10.1109/61.544265.
IEEE Guide for the Interpretation of Gases Generated in Mineral Oil-Immersed Transformers. IEEE Std C57.104-2019 (Revision of IEEE Std C57.104-2008), 2019, 98 p. doi: https://doi.org/10.1109/IEEESTD.2019.8890040.
Bouchaoui L. Diagnostic des transformateurs de puissance par la méthode d'analyse des gas dissous : application des réseaux de neurones. Algeria, Université Ferhat Abbas Sétif 1 Publ., 2010. 155 p. (Fra). Available at: https://mmagister.univ-setif.dz/images/facultes/TEC/2010/BOUCHAOUI%20Lahcene.pdf (accessed 20 July 2020).
Fofana I. Power transformer diagnostics, monitoring and design features. Switzerland, MDPI Publ., 2018. 254 p. Available at: https://www.mdpi.com/books/pdfview/book/1073 (accessed 20 July 2020).
Paul D., Sen P., Goswami A.K. A probabilistic approach of fault detection through dissolved gas analysis in transformer and reactor. 2020 IEEE International Conference on Power Electronics, Smart Grid and Renewable Energy (PESGRE2020), 2020, pp. 1-6. doi: https://doi.org/10.1109/pesgre45664.2020.9070495.
Taha I.B.M., Mansour D.-E.A., Ghoneim S.S.M., Elkalashy N.I. Conditional probability-based interpretation of dissolved gas analysis for transformer incipient faults. IET Generation, Transmission & Distribution, 2017, vol. 11, no. 4, pp. 943-951. doi: https://doi.org/10.1049/iet-gtd.2016.0886.
Illias H.A., Chai X.R., Abu Bakar A.H., Mokhlis H. Transformer incipient fault prediction using combined artificial neural network and various particle swarm optimisation techniques. PLOS ONE, 2015, vol. 10, no. 6, p. e0129363. doi: https://doi.org/10.1371/journal.pone.0129363.
Zhang W., Yang X., Deng Y., Li A. An inspired machine-learning algorithm with a hybrid whale optimization for power transformer PHM. Energies, 2020, vol. 13, no. 12, p. 3143. doi: https://doi.org/10.3390/en13123143.
Enriquez A.R.S., Lima S.L., Saavedra O.R. K-NN and mean-shift algorithm applied in fault diagnosis in power transformers by DGA. 2019 20th International Conference on Intelligent System Application to Power Systems (ISAP), 2019, pp. 1-6. doi: https://doi.org/10.1109/isap48318.2019.9065948.
Cover T., Hart P. Nearest neighbor pattern classification. IEEE Transactions on Information Theory, 1967, vol. 13, no. 1, pp. 21-27. doi: https://doi.org/10.1109/TIT.1967.1053964.
Seifeddine S., Khmais B., Abdelkader C. Power transformer fault diagnosis based on dissolved gas analysis by artificial neural network. 2012 First International Conference on Renewable Energies and Vehicular Technology, 2012, pp. 230-236. doi: https://doi.org/10.1109/revet.2012.6195276.
Jasim S.Y., Shrivastava J. Dissolved gas analysis of power transformers. International Journal of Electrical and Electronics Engineering Research (IJEEER), 2013, vol. 3, no. 5, pp. 1-10.
Taecharoen P., Kunagonniyomrattana P., Chotigo S. Development of Dissolved Gas Analysis Analyzing Program using Visual Studio Program. 2019 IEEE PES GTD Grand International Conference and Exposition Asia (GTD Asia), 2019, pp. 785-790. doi: https://doi.org/10.1109/gtdasia.2019.8715892.
Wang Z., Liu Y., Griffin P.J. A combined ANN and expert system tool for transformer fault diagnosis. IEEE Transactions on Power Delivery, 1998, vol. 13, no. 4, pp. 1224-1229. doi: https://doi.org/10.1109/61.714488.
Rogers R.R. IEEE and IEC Codes to Interpret Incipient Faults in Transformers, Using Gas in Oil Analysis. IEEE Transactions on Electrical Insulation, 1978, vol. EI-13, no. 5, pp. 349-354. doi: https://doi.org/10.1109/TEI.1978.298141.
IEC 60599:2015. Mineral oil-filled electrical equipment in service – Guidance on the interpretation of dissolved and free gases analysis. 2015. Available at: https://webstore.iec.ch/publication/23323 (accessed 20 July 2020).
Mellah H., Hemsas K.E., Taleb R., Cecati C. Estimation of speed, armature temperature and resistance in brushed DC machines using a CFNN based on BFGS BP. Turkish Journal of Electrical Engineering & Computer Sciences, 2018, vol. 26, no. 6, pp. 3181-3191. doi: https://doi.org/10.3906/elk-1711-330.
Da Silva I.N., de Souza A.N., Hossri R.M.C., Hossri J.H.C. Intelligent system applied in diagnosis of transformer oil. 2000 Eighth International Conference on Dielectric Materials, Measurements and Applications (IEE Conf. Publ. No. 473), 2000, pp. 330-334. doi: https://doi.org/10.1049/cp:20000528.
Mellah, H., Hemsas, K., Taleb, R. Intelligent sensor based Bayesian neural network for combined parameters and states estimation of a brushed DC motor. International Journal of Advanced Computer Science and Applications, 2016, vol. 7, no. 7, pp. 230-235. doi: https://doi.org/10.14569/ijacsa.2016.070731.
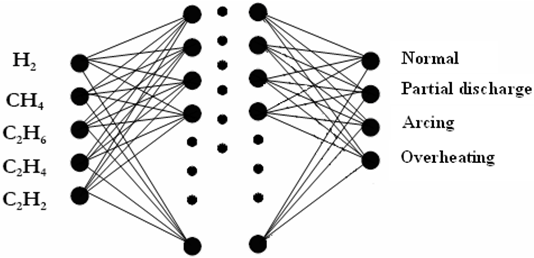
Downloads
Published
How to Cite
Issue
Section
License
Copyright (c) 2021 L. Bouchaoui, K. E. Hemsas, H. Mellah, S. Benlahneche

This work is licensed under a Creative Commons Attribution-NonCommercial 4.0 International License.
Authors who publish with this journal agree to the following terms:
1. Authors retain copyright and grant the journal right of first publication with the work simultaneously licensed under a Creative Commons Attribution License that allows others to share the work with an acknowledgement of the work's authorship and initial publication in this journal.
2. Authors are able to enter into separate, additional contractual arrangements for the non-exclusive distribution of the journal's published version of the work (e.g., post it to an institutional repository or publish it in a book), with an acknowledgement of its initial publication in this journal.
3. Authors are permitted and encouraged to post their work online (e.g., in institutional repositories or on their website) prior to and during the submission process, as it can lead to productive exchanges, as well as earlier and greater citation of published work.